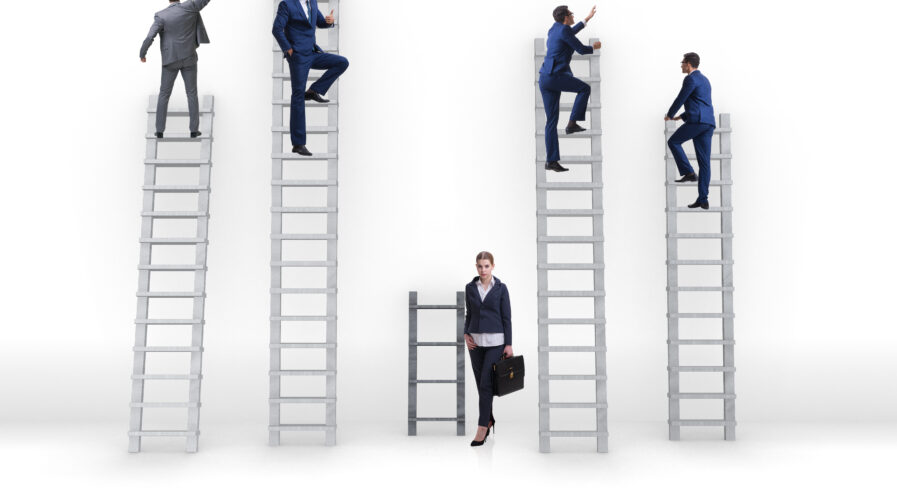
Addressing gender bias in AI. Source: Shutterstock
Can we please do more about gender bias in AI?
- The models and systems we create and train are a reflection of ourselves — including Artificial Intelligence
- There is a very real risk that, instead of solving the problem of gender bias, AI will only exacerbate it further.
It is not a coincidence that virtual personal assistants such as Siri, Alexa, and Cortana have female names and come with a default female voice. Artificial Intelligence (AI) relies on algorithms that learn from real-world data made by humans, so it can, inadvertently, reinforce gender bias.
Gartner predicts that by 2022, 85% of AI projects will deliver erroneous outcomes due to bias in data, algorithms, or the teams responsible for managing them. In order to produce technology that is fairer, there must be a concerted effort from researchers and machine learning teams across the industry to correct this imbalance.
It is also important to note that gender bias is not merely a male problem. A UNDP report entitled Tackling Social Norms found that about 90% of people — both men and women — hold some bias against women. That said, AI applications are generally trained using data that are generated by humans, and humans are inherently biased. For instance, natural language processing (NLP), a critical ingredient of common AI systems like Amazon’s Alexa and Apple’s Siri, among others, has been found to show gender biases – and this is not a standalone incident.
While most of the virtual assistants powered by AI have women’s voices, the most powerful computer in the world, Watson, is named after a man. Harvard Business Review cited word-embeddings as a bias aspect of AI. Like a game of word association, these systems can often associate ‘man’ with ‘doctor’ and ‘woman’ with ‘nurse’. These don’t reflect modern society, or at least how we want modern society to progress.
How does gender bias occur in AI?
Simply put, gender bias occurs during machine learning and an example is in the dataset. Say there are not enough women contributing, there will be holes in the AI’s knowledge, and this is why bias errors happen. Machine learning is of course led by humans, which means their own bias will be incorporated within the AI system.
The problem is, algorithms are everywhere, making decisions on our behalf in ways that are often opaque to us. With 78% of AI professionals being men, male experiences inform and dominate algorithm creation. This gender bias can have significant adverse implications for women. A real-world built and designed using data for men ignores the needs of half its population. This holds true even when artificial intelligence is harnessed to solve challenges facing all of humanity.
What can we do about it?
Frankly speaking, technologies are rarely gender-neutral in practice. If AI and automation continue to ignore women’s experiences or leave women behind, everyone will be worse off. Experts reckon that all standards related to AI and automation should integrate a gender perspective in a holistic manner, rather than treating gender as merely a bias issue to be managed.
Hence why, the first and most important step in fighting gender bias in AI is to correct the datasets used in training and testing systems, models, and algorithms. The data that informs algorithms, AI, and automation should be sex-disaggregated, otherwise, the experiences of women will not inform these technological tools and in turn, might continue to internalize existing gender biases against women. Moreover, even data related to women should be guarded against any inherent gender bias.
For that to be possible, women should be active participants—rather than mere passive beneficiaries—in creating AI and automation. Women and their experiences should be adequately integrated into all steps related to the design, development, and application of AI and automation. In addition to proactively hiring more women at all levels, AI and automation companies should engage gender experts and women’s organizations from the outset in conducting human rights due diligence.
READ MORE
- Data Strategies That Dictate Legacy Overhaul Methods for Established Banks
- Securing Data: A Guide to Navigating Australian Privacy Regulations
- Ethical Threads: Transforming Fashion with Trust and Transparency
- Top 5 Drivers Shaping IT Budgets This Financial Year
- Beyond Connectivity: How Wireless Site Surveys Enhance Tomorrow’s Business Network