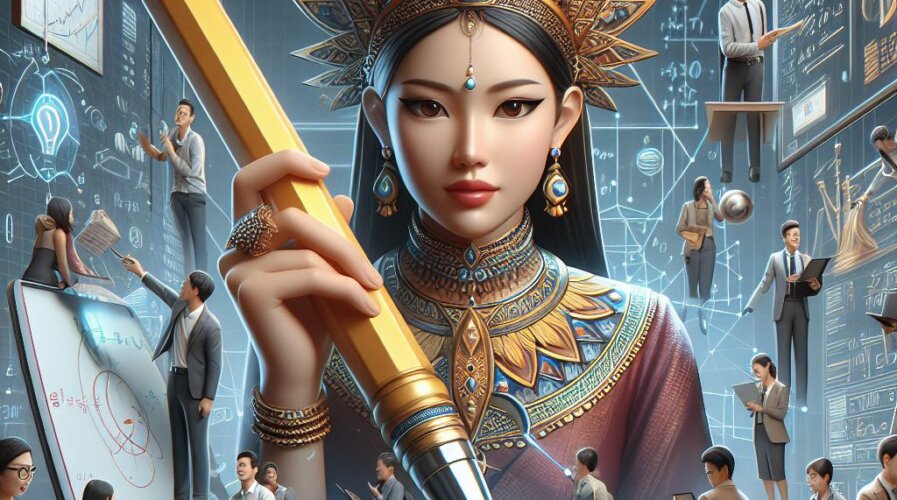
Alibaba DAMO Academy announces the launch of SeaLLMs. (Image generated by AI).
Alibaba DAMO Academy introduces SeaLLMs, inclusive AI language models for SEA
- DAMO Academy unveiled LLMs capable of processing local languages and performing tasks that better align with local customs, style and legal stipulations.
- Called SeaLLMs, the model supports Vietnamese, Indonesian, Thai, Malay, Khmer, Lao, Tagalog and Burmese.
- The models help to reflect Southeast Asia’s diverse linguistic and cultural landscape.
Last week, Singapore announced plans to develop Southeast Asia’s first large language model (LLM) ecosystem which it hopes can cater to the region’s diverse culture and languages. The concern was that most of the LLMs currently available had values and norms that catered more towards Western countries.
Just as Singapore’s LLM plans get into development, China’s Alibaba DAMO Academy has also unveiled SeaLLMs. Alibaba SeaLLMs is a pioneering LLM that comes with 13 billion parameter and 7 billion parameter versions that are specifically designed to cater to Southeast Asia’s diverse languages as well.
While English is still widely spoken in the region, most countries still have their native languages that remain an important communication tool. For example, in Malaysia, while English is predominantly spoken, Malay is still the language used by government agencies for official communications. The same applies to Thailand, Indonesia, Cambodia, Laos, Vietnam and Myanmar. In the Philippines, English is a major language, but Tagalog is mostly used in communication.
The models are expected to offer optimized support for several local languages in the region. They include Vietnamese, Indonesian, Thai, Malay, Khmer, Lao, Tagalog and Burmese. SeaLLM-chat, the conversation model, shows adaptability to the unique cultural fabric of each market, aligning with local customs, styles, and legal frameworks, working as a chatbot assistant for businesses engaging with Southeast Asia markets.
“In our ongoing effort to bridge the technological divide, SeaLLMs is a series of AI models that not only understand local languages but also embrace the cultural richness of Southeast Asia. This innovation is set to hasten the democratization of AI, empowering communities historically underrepresented in the digital realm,” said Lidong Bing, director of the language technology lab at Alibaba DAMO Academy.
Echoing this sentiment, Luu Anh Tuan, Assistant Professor in the School of Computer Science and Engineering (SCSE) at Nanyang Technological University, commented, “Alibaba’s strides in creating a multilingual LLM are impressive. This initiative has the potential to unlock new opportunities for millions who speak languages beyond English and Chinese.”
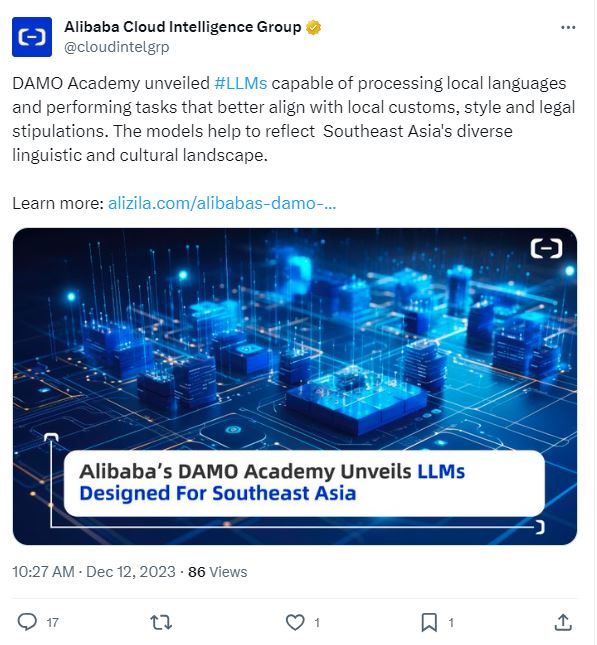
The models help to reflect Southeast Asia’s diverse linguistic and cultural landscape.
Trying out Alibaba SeaLLMs
MABA, IndoBERT, and ThaiBERT are all examples of Southeast Asian large language models. They are trained on large corpora of texts from various sources, such as Wikipedia, news articles, social media posts, and books. They can perform various natural language processing tasks, such as text classification, sentiment analysis, question answering, and text summarization.
Alibaba explained that the SeaLLMs are open-sourced on Hugging Face, with released check point and available for research and commercial use. As such, Tech Wire Asia decided to try several prompts on the Malay and Tagalog language versions of the chat.
For Malay, a basic conversation with the chatbot seemed the same as what several Malay-speaking chatbots in Malaysia are already capable of. In fact, the conversation did not feel as natural as it would, but the model is still learning and is definitely able to improve. We also prompted a few Malay slang terms to the model to see if it could understand the local dialect. Unfortunately, the LLM is – at least for you – unable to do so.
For example, we prompted in a local dialect on where a good place would be to get “satay” in Kajang (a town famous for the dish in Malaysia). The LLM was unable to provide information about the restaurant but instead wanted to give directions to the restaurant – something could have gone wrong with the translation. Again, the model is still learning and we believe it will only get better over time.
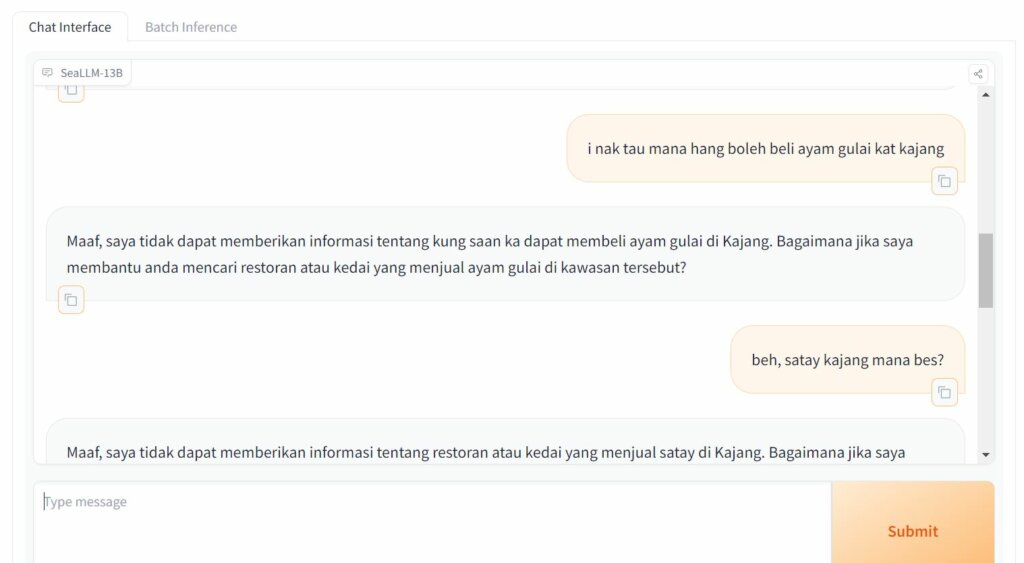
TWA tried some Malay prompts.
Meanwhile, for Tagalog, while none of us at TWA speak the language, we used Google Translate to get some prompts and the results were interesting. Overall, Alibaba may have a solution that could be a game-changer, especially for businesses looking to utilize chatbots. The responses were generated rather fast and even though some prompts were not entirely accurate, the LLM could still get the gist of the message.
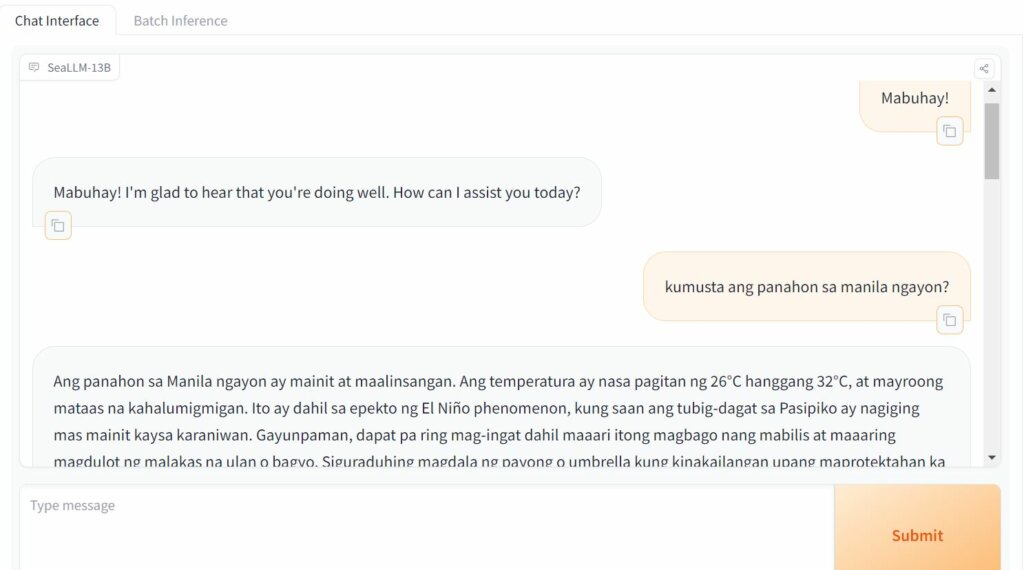
TWA also prompted Tagalog.
How does SeaLLM work?
According to a report by Alibaba DAMO researchers, SeaLLM-base models underwent pre-training on a diverse, high quality dataset inclusive of the languages from the region. This is to ensure a nuanced understanding of local contexts and native communication.
“The pre-training dataset of SeaLLMs is formed by documents from diverse public sources, including web texts (eg, Common Crawl), news documents (eg, CC-News), academic articles, and texts with expert knowledge (eg, Wikipedia). We first employ FastText language identifier to filter out the documents that do not belong to SEA languages. To further remove harmful or undesirable content, we develop a pipeline with various data cleaning and filtering modules to preprocess the collected data. Meanwhile, to maintain the English performance of SeaLLMs, we also introduce a set of high-quality English texts sampled from RedPajama-Data into pre-training,” researchers said.
This foundational work lays the groundwork for chat models like SeaLLM-chat models, which benefit from advanced fine-tuning techniques and a custom-built multilingual dataset. As a result, chatbot assistants based on these models can not only comprehend but respect and accurately reflect the cultural context of these languages in the region, such as social norms and customs, stylistic preferences and legal considerations.
A notable technical advantage of Alibaba SeaLLMs is their efficiency, particularly with non-Latin languages. They can interpret and process up to nine times longer text (or fewer tokens for the same length of text) than other models like ChatGPT for non-Latin languages such as Burmese, Khmer, Lao, and Thai. That translates into more complex task execution capabilities, reduced operational and computational costs, and a lower environmental footprint. Note: TWA is unable to verify this but native speakers are welcome to give it a try and see how it works.
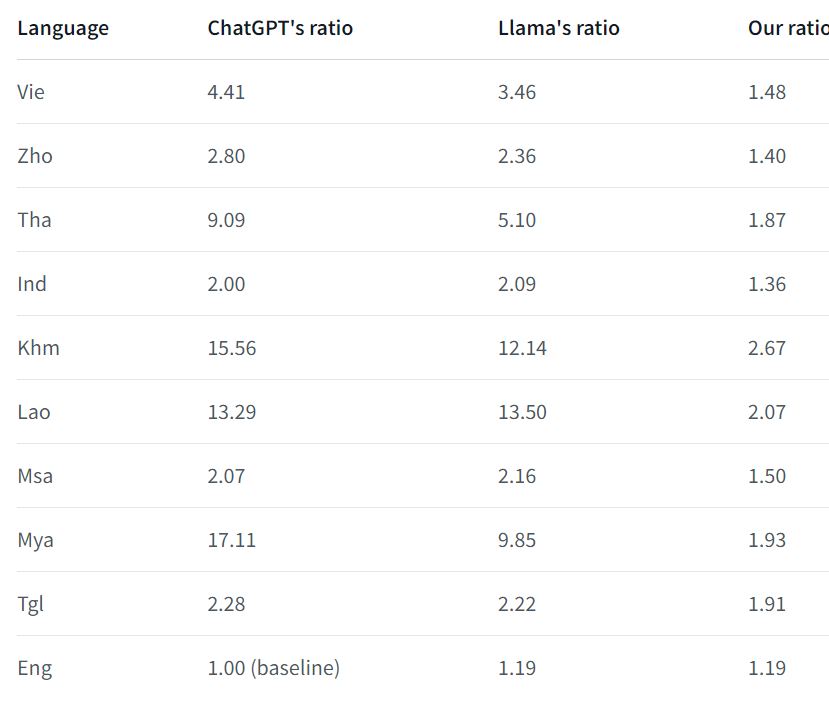
SeaLLM can interpret and process up to nine times longer text.
Alibaba also boasts SeaLLM’s 13 billion parameters. It claimed that SeaLLM-13B outshines comparable open-source models in a broad range of linguistic, knowledge-related, and safety tasks, setting a new standard for performance. When evaluated through the M3Exam benchmark (a benchmark consisting of exam papers from primary school to university entrance examination), SeaLLMs display a profound understanding of a spectrum of subjects, from science, chemistry, and physics to economics, in Southeast Asia languages, outperforming its contemporaries.
“SeaLLM-13b models exhibit superior performance across a wide spectrum of linguistic tasks and assistant-style instruction-following capabilities relative to comparable open-source models. Moreover, they outperform ChatGPT-3.5 in non-Latin languages such as Thai, Khmer, Lao, and Burmese,” the report said.
The SeaLLMs also excel in the FLORES benchmark. The FLORES benchmark assesses machine translation capabilities between English and low-resource languages—those with limited data for training conversational AI systems, such as Lao and Khmer. They surpass existing models in these low-resource languages and deliver performances on par with state-of-the-art (SOTA) models in most high-resource languages, such as Vietnamese and Indonesian.
READ MORE
- Data Strategies That Dictate Legacy Overhaul Methods for Established Banks
- Securing Data: A Guide to Navigating Australian Privacy Regulations
- Ethical Threads: Transforming Fashion with Trust and Transparency
- Top 5 Drivers Shaping IT Budgets This Financial Year
- Beyond Connectivity: How Wireless Site Surveys Enhance Tomorrow’s Business Network